Thanks html-midi-player for the MIDI visualization.
Introduction
In this paper, we employ a two-stage Transformer-based model on emotion-driven piano performance generation, considering the inadequate emotion modeling through end-to-end paradigms in previous works. The first stage focuses on valence modeling via lead sheet (melody + chord) composition , while the second stage addresses arousal modeling by introducing performance-level attributes, such as articulation, tempo, and velocity.
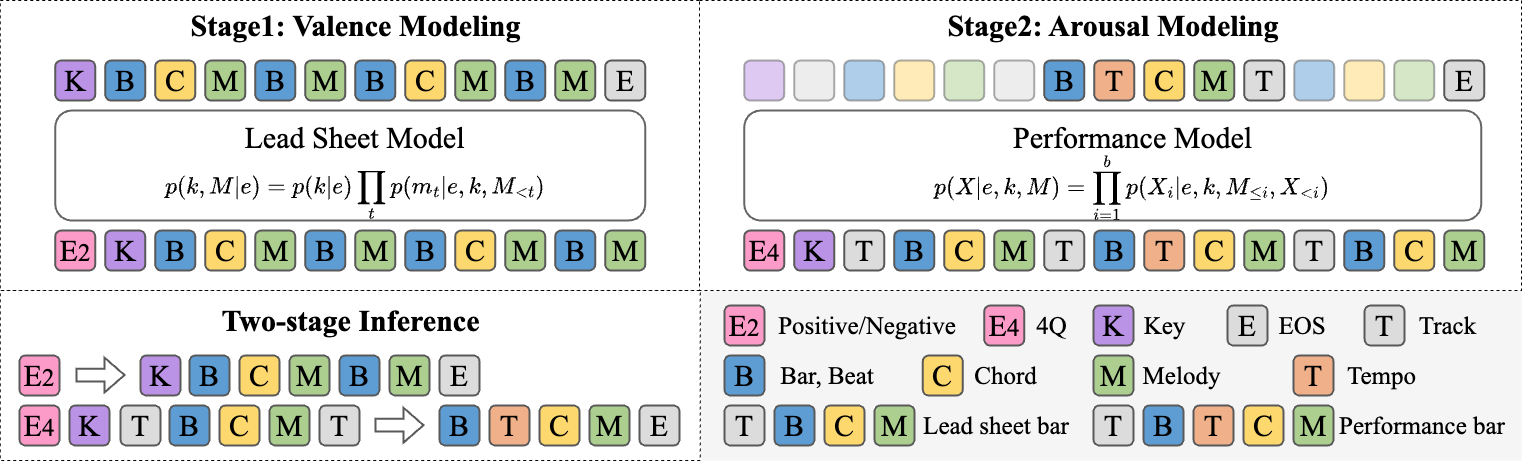
To further capture features that shape emotional valence, we propose a novel functional representation for symbolic music, designed as an alternative to REMI, a popular event representation that uses note pitch values and chord names to encode symbolic music.
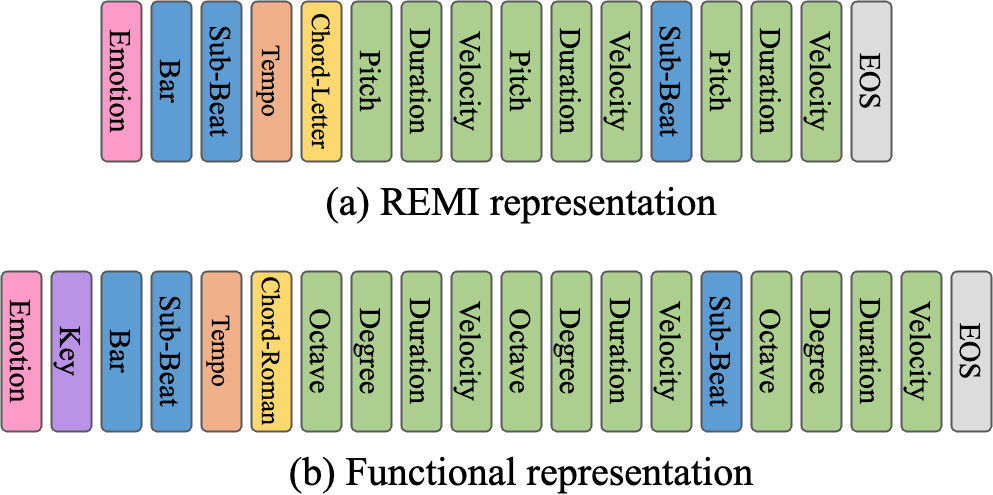
This new method takes musical keys into account, recognizing their significant role in shaping valence perception through major-minor tonality. It encodes both melody and chords with Roman numerals relative to musical keys, to consider the interactions among notes, chords and tonalities.
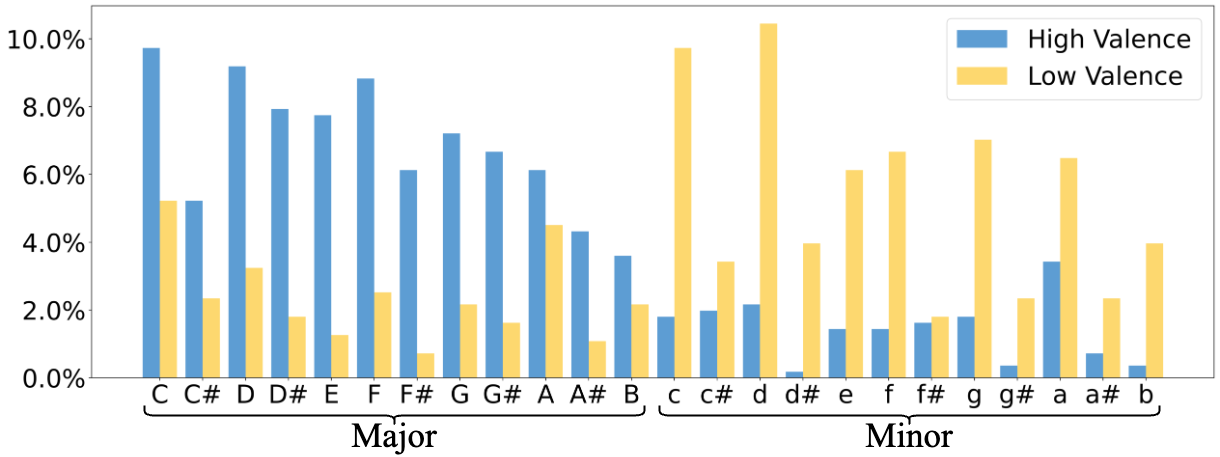
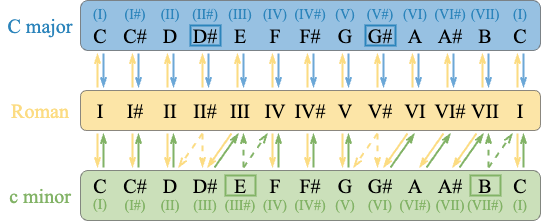
Experiments demonstrate the effectiveness of our framework and representation on emotion modeling. Additionally, our method enables new capabilities to control the arousal levels of generation under the same lead sheet, leading to more flexiable emotion controls.
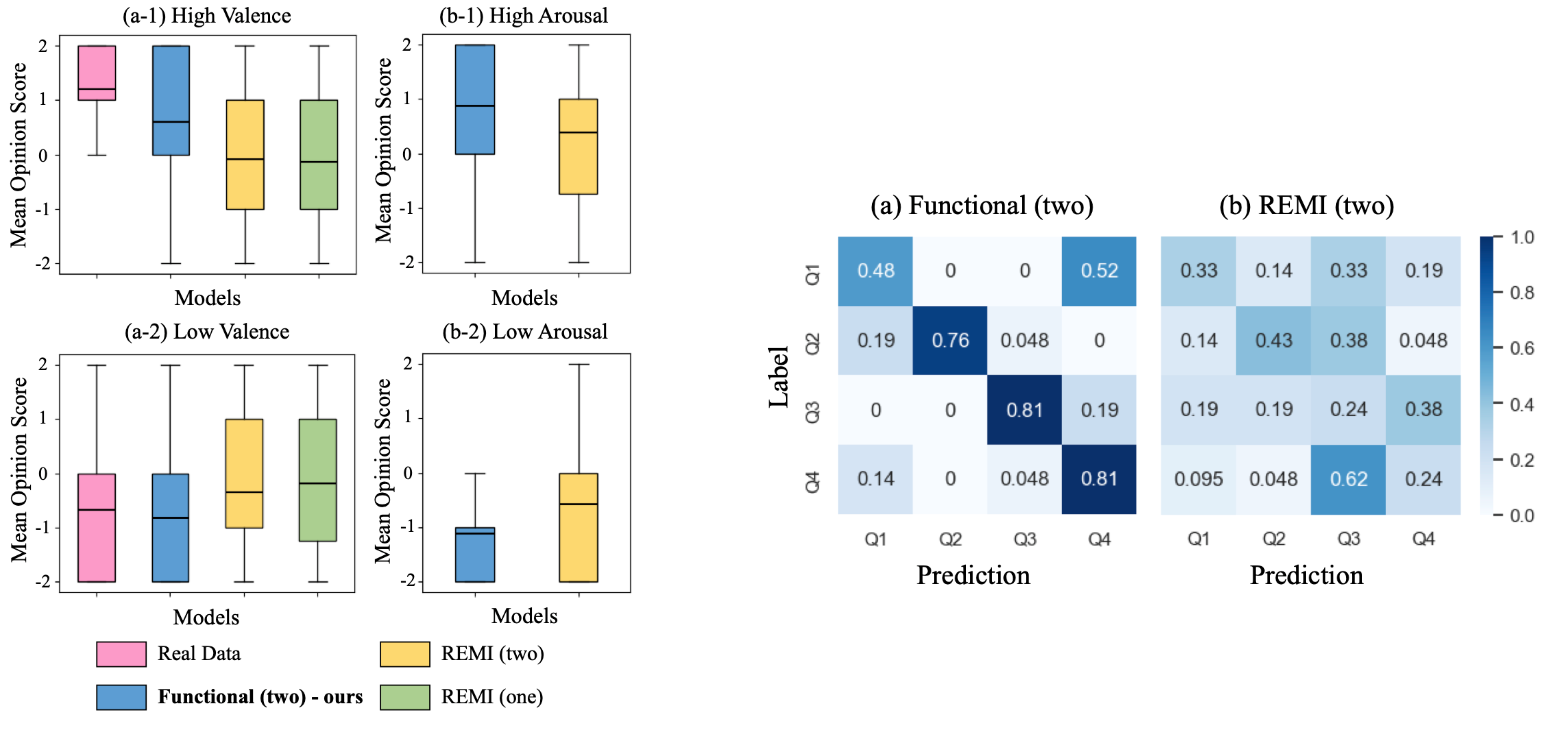
Generation Samples
We show some generation samples below from three models:
- REMI (one): one-stage generation model with REMI representation, baseline
- REMI (two): two-stage generation model with REMI representation, one variant of our proposed framework
- Functional (two): two-stage generation model with functional representation, our main proposal
Same Lead Sheet, Different Arousal Performance
This section presents piano performances with different arousal levels (Low Arousal, High Arousal) that were generated in the second stage of our framework, based on the same lead sheet (Lead Sheet) produced in the first stage. This is a new emotion-based music generation application with our two-stage framework, either with REMI or functional representation.
Examples with positive valence
Given Lead Sheet | High Arousal | Low Arousal | |
---|---|---|---|
REMI (two) | |||
Functional (two) | |||
Examples with negative valence
Given Lead Sheet | High Arousal | Low Arousal | |
---|---|---|---|
REMI (two) | |||
Functional (two) | |||
4Q generations
This section shows generated examples for each Quadrant. It was found that REMI representation has poor performance in valence modeling based on our user study.
Q1 (High Valence, High Arousal)
REMI (one) | |||
REMI (two) | |||
Functional (two) |
Q2 (Low Valence, High Arousal)
REMI (one) | |||
REMI (two) | |||
Functional (two) |
Q3 (Low Valence, Low Arousal)
REMI (one) | |||
REMI (two) | |||
Functional (two) |
Q4 (High Valence, Low Arousal)
REMI (one) | |||
REMI (two) | |||
Functional (two) |
Authors and Affiliations
-
Jingyue Huang
PhD student @ UC San Diego
jih150@ucsd.edu -
Ke Chen
PhD student @ UC San Diego
knutchen@ucsd.edu -
Yi-Hsuan Yang
Professor @ National Taiwan University / Joint-Appointed Researcher @ Academia Sinica
yhyangtw@ntu.edu.tw, affige@gmail.com